What Is Deepfake Technology?
Deepfake is a digital media technique in which a user literally takes original text, image, video, or audio and then manipulates it to appear like someone else utilizing an advanced neural network and artificial intelligence technologies.
Deep-fake technology has developed from the chicanery of an innocent computer geek to a sinister slandering tool, since its first appearance a few years ago. In this post, we ‘re going to see just what this dreaded deep-fake software is, how it operates, what different types it comes in, and how we can identify or debunk a deep-fake.
What is Deepfake?
Deepfake is one of the buzzwords of media technologies in which a person literally takes existing text, image, video, or audio and then manipulates it to appear like someone else utilizing an advanced neural network and artificial intelligence technologies.
Do you want to insert offensive language in your nemesis ‘s mouth? Or change your favourite Hollywood celebrity to the movie protagonist? Maybe just want to be spinning with Michael Jackson? Then what you need is deep-fake!
Deep-fake content is dramatically on the rise. Unfortunately, deep-fake software has been employed on a variety of occasions to achieve electoral leverage, tarnish a rival ‘s reputation or commit financial fraud.
Let’s look now at the three major forms of deep-fakes and discuss the theory of data that helps them to function. They would also concentrate on deep-fake identification techniques that are being utilized by developers and technology experts to prevent fraudulent usage of deep-fakes.
Deepfake text
During the early days of artificial intelligence and encoding of natural languages (NLP), an artistic task such as drawing or writing presented a problem for a computer. However, in 2020, the high standard of AI-generated prose with humanistic zeal and accuracy can now be written with powerful language models and libraries developed over the years through the work of researchers and practitioners in the field of data sciences.
GPT-2
Take GPT-2, for example, the new breed of a Silicon Valley OpenAI Research Laboratory text-generation program. The layman and domain experts have been struck by the technology ‘s ability to channel coherent text with minimum leads.
Over 8 million text documents were scrapped (the relevant data extraction method for web pages), combined with the GPT-2 AI modelling and training parameters, were used by OpenAI engineers.
The essence of profound technology such as deep learning, which uses artificial technology, is that the software is trained to think and to adapt using past data fed by data sets.
You can simply punch into the title with the GPT-2 and create a fictitious story about that title with the fabricated text algorithm. Or only deliver the first line of a poem, and the entire verse will be returned.
Many media outlets use profound algorithms and web scrapping to produce stories or blogs that the program itself publishes.
Researchers at the Center for Terrorism, Extremism and Counter-terrorism (CTEC) at the Middlebury Institute of International Studies warn that tools such as GPT-2 can be used in misusing racial predominance or disseminating radical messages from extremist organizations.
Deepfakes on social media
Deepfake technology can also be used to create a false online identity in conjunction with writing stories or blogs that would be difficult for a regular user to distinguish. For instance, on social networking sites like LinkedIn and Twitter, a Bloomberg (non-existing) journalist named Maisy Kinsley was a plausibly profound figure. Her profile image seemed odd, probably generated by a computer. Perhaps for financial gain, as Maisy Kinsley ‘s profile has tried regularly to link to Tesla’s short sellers in social networks.
Short-sellers are people on the stock market who are bearish and short, i.e. sell the stock in the expectation that the stock price will drop and then buy stocks at lower prices to make a good profit effectively.
Another profile named Katie Jones, who allegedly mentioned working at the Center for Strategic and International Studies, was found to be deep-fake spying with the mala fide intention.
Detecting textual deepfakes
The software tool Grover was created by researchers from the Institute of Artificial Intelligence, Allen, to detect digital contents floating online. Researchers claim that deep-written texts can be detected 92% of the time by this software. Grover is working on an open-source web archive test collection compiled by Common Crawl, a crawler. Similarly, a Harvard scientist team and the Watson MIT-IBM laboratory have come together to create a Giant Language Model Test Room, a web-based platform designed to test the generation of the input text by AI.
Deepfake video
The main arsenal of deepfakes is to produce fake pictures and videos. It’s the deepest type because we live in the omnipresent world of social media, where pictures and videos explain events and stories better than plain text.
Modern video generation AI is more powerful than the natural language of its predecessors and therefore more threatening. Hyperconnect, Seoul-based technology company, recently developed a tool called MarioNETte which can produce profound videos of historical figures, celebrities and politicians. This is done by another individual, whose facial expressions are then overlaid with the targeted personality whose deepfake is to be created.
How deepfake video is produced?
A technique known as generative opposed networks (GAN) is used in this video trickery. GAN is part of the neural networks apprenticeship group. These networks are intended to emulate the human brain’s neuronal processes. Programmers can train neural networks for a certain task to recognize or control.
Two neural networks are set up to produce a reasonable output in GANs for a deep generation. The goal is to make sure that the depths are produced so that they look as real as possible. The core of the GAN is the rivalry of the two neural networks. In GAN, the picture forger and the falsifying detector frequently try to outsmart each other. The same data set is used to train both neural networks.
The first network is the generator whose job is to produce a forged image that is as realistic as possible using noise vector (a list of random numbers). The second network, the discriminator, determines the truthfulness of the images generated. It compares the generator’s forged image to the actual images in the dataset to determine which images are real and which are fake. The generator varies the parameter for image generation based on these findings. The process continues until the discriminator figures out that a created image is fake and is then used in the final result. This is the reason deepfakes look so shockingly real.
Detecting deepfake videos
Worldwide forensic specialists work hard to find ways and instruments to locate deep fakes, as they are increasingly persuasive on a daily basis.
Take this profound demonstration video of Obama published in 2018 by Buzzfeed that astounded viewers all over the world. You may look at it, here’s a link: https://youtu.be/cQ54GDm1eL0
As machine learning tools meet the market, producing credible counterfeit videos that could be used to spread propaganda-led news or simply harass a targeted person has become easier.
The US Department of Defense (DARPA) has developed a device called Media Forensics to spot deep-fakes. The programme, initially designed to automate existing forensic tools, used AI to counteract AI-driven deepfakes with the rise of deepfakes. Let’s look at how it’s going to work.
The resulting video produced with deepfake technically differs significantly from the original way metadata is distributed. These differences are called matrix glimpses, which is the purpose of DARPA deeper detection tool for detection of deepfake media.
Siwei Lyu, a professor at the State University of New York’s Department of Computer Science, has noted that faces are seldom blinked using deep-fake technology. That seems unnatural, even if they do. He posits this is because the bulk of deep-fake videos are equipped using still photographs. And then, a person’s photos are normally taken while their eyes are open. Many data points on facial expressions, such as lifting the upper lip during the speech, whether they raise their heads, etc. will also offer hints as to whether the streaming footage is unreal.
Deepfake audio
The power of artificial intelligence and neural networks is not confined to text, images, and video alone. With the same ease, they may clone a person’s voice. All that’s needed is an audio recording data set of a person whose voice needs to be emulated. Deep-fake algorithms learn from the set of data and are inspired to replicate the prosody of the speech of a targeted individual.
Commercial software is being released on the market, such as Lyrebird and Deep Voice, where you only need to speak a few sentences before your voice and intonation become accustomed to the AI. As you feed into more of your audio, that software becomes powerful enough to clone your voice. You can only send a sentence or a paragraph after feeding your audio samples in the dataset and this deepfake app can narrate the text in your voice!
Identifying deepfake audio
There aren’t many specialized deep-fake audio applications available right now, but researchers and technology companies are working in this area to come up with improved defensive solutions.
For instance, developers at tech startup Resemble last year developed an open-source tool called Resemblyzer to detect deep-fake audio clips. Resemblyzer uses sophisticated machine-learning algorithms to generate representations of voice samples from computations to determine whether they are true or false. Whenever a user submits an audio file for review, a mathematical representation is created which summarizes the specific characteristics of the voice sample submitted.
The advancement of deepfakes
Last year, an investigation undertaken by Deeptrace laboratories found that more than 14,000 deep-fake videos lurk online. They also noted an 84% jump in their output in just seven months. Interestingly, more than 90% of deep-fake images are sexual content in which famous women are transformed facially into porn.
As deep-fake is gaining considerable momentum, it raises a serious question of intruding not just on anonymity, but also on human integrity. Ironically, artificial intelligence itself is being used to combat AI-powered deepfakes.
Although a ‘good’ AI helps to distinguish deep-fakes, this detection system relies heavily on the dataset that it absorbs for testing. This means they can work perfectly to spot celebrities’ deep-faced images because there is a huge amount of data on them. But it will be difficult for these identification systems to detect the deep-fake of a person who has a low profile.
Social media technology giants also work on deep-fake detection systems. Facebook recently announced it is working on an automated system to identify and weed out deep-fake content on its platform. On similar lines, Twitter has proposed flagging and eliminating deep-fakes if they are found to be provocative.
While we appreciate and respect these tech firms’ attempts, it is only time that can tell how successful they are in keeping malicious deep-fakes away!
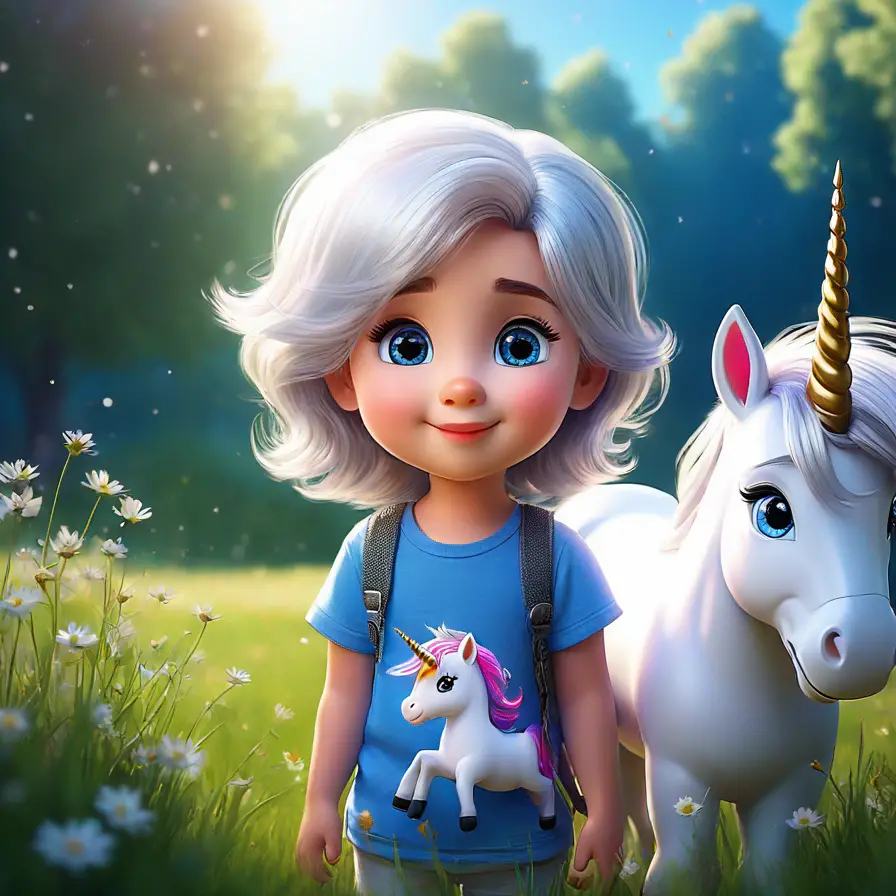
Hi, I’m Jodie! I’m a spain-Moroccan writer with a passion for imagination, adventures, magic and stories with heart.
Please don’t hesitate to contact me for any questions, suggestions, comments or feedback.
Add comment